Oct 04,2023
With the explosion of “Gen AI” over the past few months, and the wide ranging debate on it’s pros and cons, we conducted a deep-dive into the subject and are happy to share our findings.
This is the first of a three part series that explores the potential environmental, social and governance (ESG) impact of Generative AI, the potential mitigation strategies to address the ESG concerns and the current state of regulations vis-à-vis the above. This first part focuses on contextualizing the ESG impact.
While both AI and ESG are highly technical and complex areas, we have tried to keep it as simple as possible while incorporating the key technical nuances.
Natural Language Processing (NLP), Natural Language Understanding (NLU) and Natural Language Generation (NLG) – that has always been the expected progression of language based AI models. NLG which is now more commonly known as Generative AI or GenAI well and truly became a part of public discourse with the public launch of ChatGPT by OpenAI in November 2022. Built on Large Language Models (LLMs), this represents a major step forward in the quest of Artificial General Intelligence (AGI).
LLMs represent a quantum leap in the age of digital transformation by rethinking the parameters of human-machine interaction. These revolutionary AI systems are incredibly accurate and complex when it comes to processing and understanding (read contextualizing) human language and generating responses in the same vein. These advanced capabilities are the outcome of consuming vastly higher amounts of data as well as running more sophisticated and computationally intensive inference models – both of which come at a cost.
In that context, we must examine the effects of LLMs from an environmental, social, and governance (ESG) viewpoint while we embrace the potential advantages of LLMs. At Morphosis, given our focus on investing in AI as well as ESG positive businesses, it is imperative to explore the complex interplay between LLMs and ESG, illuminating how businesses might effectively and ethically use the power of these models in our digital economy.
The below figure shows the AI development lifecycle and the E, S & G concerns associated with each stage of the value chain:
Note:
* There are other considerations from an ESG perspective but have been omitted here in the interest of focusing on the major concern areas
* There is a reasonable degree of overlap between the S & G related areas of concern and the above classification of certain concern areas is fungible across S & G
Environmental view of LLMs
LLMs are among the biggest ML models, spanning up to hundreds of billions of parameters, requiring millions of GPU hours to train, and emitting carbon in the process. As these models grow in size – which has been the trend in recent years – it is crucial to understand to also track the scope and evolution of their carbon footprint.
“Generative AI models offer impressive outputs but demand high computing power and a massive carbon footprint. Managing their inference costs is a significant barrier for organizations seeking to adopt generative AI on a larger scale”, – Yonatan Geifman, Ph.D, CEO of Deci
As the name suggests, LLMs consume large volumes of data that is multiple orders of magnitude larger than conventional AI models. For example, GPT-3 (the engine on which ChatGPT runs) was trained on 175 billion parameters as compared to a traditional AI chatbot which is trained on anywhere between 1 million to 25 million parameters (based on an analysis of the most commonly used datasets for training customer support chatbots) i.e. 7,000 to 175,000 times higher volume of data.
The below graphic shows the sheer size of the most popular foundational models in use today:
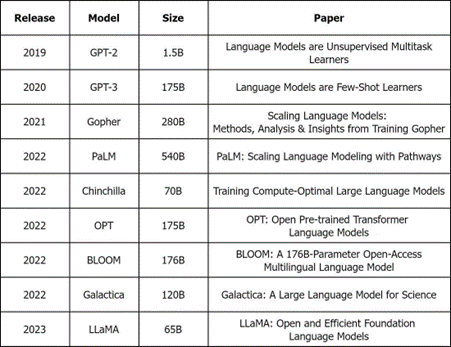
Given the sheer volume of data required for building and training these models, the process of finding the right data and ingesting it in the required format is highly computationally intensive. Add to that the infrastructure required to train the model – in terms of GPUs deployed, and the associated energy consumption – and the total carbon footprint of LLMs quickly adds up. Even before it has been deployed address an real world use case. A simple estimate of the carbon emission can be done based on the below formula:
(Total energy consumed to train the model) x (Carbon intensity of the grid) x (Adjustment factor)
Where the adjustment factor is a constant to account for the share of energy used by other infrastructure such as storage, pre-processing etc. which is essential to the model development but not accounted for in the training energy consumed considered above. The Adjustment factor is ~ 1.85 x
Based on the above, the carbon footprint to train all the models listed above is ~ 2.8 million tonnes of CO2 (assuming training time is directly proportional to the model size i.e. number of parameters and extrapolated from exact numbers available for BLOOM). While this may not seem like a very high number, all AI models undergo data drift and concept drift and must be consistently re-trained on updated data. While re-training is not as computationally intensive, the larger the model, more often is the re-training need. Indeed, ChatGPT for all its positive reception, is already facing serious issues with model performance.
Assuming a 10% drift every quarter, and a directly proportional cost of retraining, all the models above would contribute an additional ~1.1 million tonnes of CO2 every year just to maintain performance levels.
While training costs are high, estimates show that deployment and inference costs are significantly higher. One particular research has estimated that every query to an LLM model via APIs generates ~ 1.5 grams of CO2. Extrapolating this to Google’s search business that receives ~ 1.2 trillion queries annually, an 100% LLM powered engine would generate ~ 1.8 million tonnes of CO2.
This serves to contextualize that as LLMs are deployed and usage increases, we would see an exponential rise in their carbon footprint and must work towards finding ways to mitigate this. You can read more about potential mitigation strategies in Part 3 of this series.
Social & Governance view of LLMs
Given the deeply interlinked nature of the Social and Governance concern areas vis-à-vis LLMs, it makes sense to explore them together rather than as separate entities. The key areas of concern in the context are Privacy, Fairness, Explainability and Accountability. These concerns are also at the forefront of all proposed regulation around AI. You can read more about it Part 2 of this series. Each of the above concerns is outlined in greater detail below. You can read more about potential mitigation strategies in Part 3 of this series.
Privacy
“Garbage in, Garbage Out” – that is the golden rule of AI. The success of any AI model depends the quality of data used to train the model. And an LLMs superior capabilities come from its ability to consume and process vastly higher amounts of data as compared to conventional AI models. This makes access to high quality data quite valuable for AI companies.
However, data ownership and privacy in the digital realm are, at best, gray areas with Big Tech companies having carte-blanche in terms of what they do with the data at their disposal. As tenuous as the legality of leveraging customer data for building LLMs is (as Meta has done with LLaMa), Companies in this space have gone further – OpenAI trained the original GPT-3 model on data scrapped from the Internet from platforms such as Twitter, Reddit and Wikipedia in addition to other sources such as books and blogs, all without seeking consent of the data creators / owners. A step that has resulted in multiple lawsuits for violating privacy, infringing copyright and “stealing data”.
On the deployment and inference side, there have been concerns of Tech companies leveraging IP owned by third parties without fair compensation. The Screen Actors Guild in the US recently went on strike to protest such a move by certain production houses .
Without a well-defined data governance framework in place with regards to how AI companies access and use data, data privacy concerns will continue to persist.
Fairness
“Bias in, Bias Out” – this is unfortunately a much less known axiom of AI and in some ways one to be much more concerned about. AI applications at their core are statistical models, albeit sophisticated ones. At a very basic level, they rely on training data to learn about a particular problem and apply those learnings to new data to deliver results. But training data – often taken from real world sources such as historical records or the internet – contains many of the biases that exist in the real world.
For example, an AI model to assess job applications could learn that historically most people in a particular job are male and end up ranking male applicants higher than their female counterparts for future openings. The solution here is simple, for data scientists to remove the gender variable while training the model – but this needs to be consciously done or we would have a highly biased and unfair AI system that would lead to increased inequality in society.
Examples of biased AI are manifold. An MIT study as early as 2017 showed that AI based predictive policing tools can be racist.
Explainability
LLMs are also known as zero shot learners i.e. unlike conventional AI models, LLMs can accomplish tasks they weren’t specifically trained for. While this opens up a myriad of possibilities, it also opens up the outcomes to be erroneous due to inaccurate logic the LLM might have applied. Logic that the LLM believes to be true but which does not apply to the problem at hand. Also referred to as “hallucination” (Which is the equivalent of making things up or lying) – it is a major area of concern when it comes to adopting complex AI systems such as LLMs.
Indeed, in June this year, a lawyer in Columbia used ChatGPT to file a plea with the federal courts. The plea cited at least six other cases to show precedent in favour of their case but the court found that the cases didn’t exist and had “bogus judicial decisions with bogus quotes and bogus internal citations,” leading a federal judge to consider sanctions. What’s more, a member of the same legal team upon revealing he had used ChatGPT to conduct legal research for the court filing that referenced the cases insisted that the artificial intelligence tool assured him the cases were real.
Such situations could occur in any realm, from transport to education to healthcare – making it extremely critical for users of the AI systems to be able to see, understand and audit the decision making process followed by the AI system
Accountability
AI accountability means explaining, validating, and taking responsibility for an AI system outcomes and is a key component of AI governance. As AI technologies become more sophisticated and autonomous, such as with LLM’s it is crucial to ensure that there are mechanisms in place to hold the relevant stakeholders accountable for the AI system's actions and outcomes.
This becomes critical as AI systems come to be used in positions of power and are relied upon for critical decision making that can impact the lives of people and society as a whole. for example, an AI system used in healthcare that is not accurate or reliable could result in incorrect diagnoses and potentially harm patients. As discussed above, issues with privacy, fairness and explainability are core to AI systems and without accountability, how do we determine who is responsible for these issues and how they can be addressed?
In the healthcare example above, consider a scenario where a hospital is reliant on LLM based AI systems to capture L1 data (i.e. basic diagnostic questions) before parsing it through another AI system to gauge criticality and queue patients accordingly. There are multiple ways in which the system could fail and the point of failure could be due to faulty training data, poorly designed algorithms or inherent flaws in the optimization problem as articulated by the stakeholders at the hospital. In the event of patients suffering because of the system’s failure, there needs to be a way to hold the parties at fault accountable. This will be critical to build trust in using AI systems.
Morphosis View on ESG implications of LLMs
We at Morphosis believe that AI systems will continue to get more sophisticated over time and that LLMs are another step in the direction of Artificial General Intelligence (AGI). With this trend, we should expect to see more computationally expensive and data hungry AI systems going forward. As such, there is tremendous business value to be derived from deploying systems but there must be a well-defined business case that includes the increased environmental impact in making the ROI assessment.
Additionally, there is a need for well-defined governance frameworks at a regional and global level to mitigate the social impact concerns of LLMs and other sophisticated AI systems. We have put together a quick read on the current state of AI regulations as well as the approach of various governments and industry bodies towards AI governance.
Keep an eye on this space for Parts 2 & 3 of this series which will be coming soon!